EECS351 STEM SEPARATION
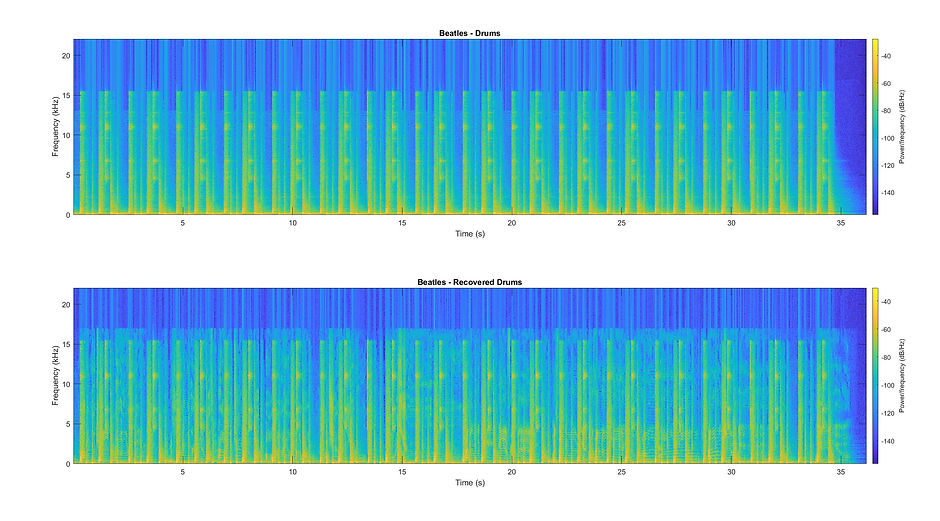
Stem Separation
EECS 351 Final Project by Surar Al-Gaylani, Carl Gerisch, James Kennedy and Alex Wildner
Source separation is the process of taking a mixture of sounds and decomposing it into individual components. For example, we might be interested in taking a mixture of several simultaneous conversations and extracting the voices of individual speakers. When source separation is applied to speech signals in this fashion, it is often referred to as the cocktail party problem. The brain proves to be remarkably adept at solving the cocktail party problem. Humans are able to filter out background noise, isolate individual speakers, and change focus from one speaker to another on the fly. For a computer, this problem proves to be rather tricky. The contributions of individual speakers may overlap in the time and / or frequency domains, which makes extracting a specific speaker difficult.
Stem separation is the application of source separation to music. Music is a mixture of sounds from different instruments including vocals, drums, bass, piano, guitar, violin, and others. We might be interested in taking a song and isolating the contributions of a specific instrument, often referred to as a stem. Stem separation proves to be especially difficult for computers, even more so than normal source separation, because the sources in music are highly correlated. In other words, overlap in the time and frequency domains for different instruments is especially pronounced. Different instruments frequently start and stop notes at the same time. Different instruments may also harmonize or play the same notes.
Despite these difficulties, stem separation is a very appealing problem to solve. There are many reasons we might want to recover the individual stems for a given song. For example, we might want to remix or rebalance the stems to amplify a particular instrument or attenuate another. We might want to extract the drums stem to determine the tempo or rhythm of the song. We might want to extract the vocals stem to perform natural language processing and identify the lyrics. We might want to extract the bass stem so that someone can learn how to play along. These are just a few examples of signal processing tasks for which source separation is an important preprocessing step. Alternatively, source separation may be an end in itself.
For our project, we have decided to tackle a simplified version of stem separation. Specifically, our goal is to create a program that receives a mixture of vocals, drums, and bass and returns individual vocals, drums, and bass stems. We’ve decided to focus on these three instruments in particular because these instruments exhibit various characteristics that make them distinct from one another in the time and / or frequency domain. Moreover, our dataset consists of a number of songs where for each song, we have access to the ground truth vocals, drums, and bass stems. Additionally, limiting the scope of our project to only these three instruments seems reasonable for a semester-long project.